近日,我院教师研究论文被IEEE J-STARS(IF: 4.715)期刊录用。论文题目是MDFENet: A Multi-scale Difference Feature Enhancement Network for Remote Sensing Change Detection(DOI 10.1109/JSTARS.2023.3260006),以下是论文的简要分享:
Abstract
The main task of remote sensing change detection (CD) is to identify object differences in bitemporal remote sensing images. In recent years, methods based on deep convolutional neural networks (CNNs) have made great progress in remote sensing CD. However, due to illumination changes and seasonal changes in the images acquired by the same sensor, the problem of ”pseudo change” in the change map is still difficult to solve. In this article, in order to reduce “pseudo changes”, we propose a multi-scale difference feature enhancement network (MDFENet) to extract the most discriminative features from bitemporal remote sensing images. MDFENet contains three procedures: first, multi-scale bitemporal features are generated by a shared weighted Siamese encoder. Then features of each scale are fed into a difference enhancement module to generate refined difference features. Finally, they are combined and reconstructed by a decoder to generate change map. The difference enhancement module includes multiple layers of difference enhancement (DE) encoder and transformer decoder. They are applied to features of different scales to establish long-range relationships of pixels semantic changes, while high-level difference features participate in the generation of low-level difference features to enhance information transmission among features of different scales, reducing “pseudo changes”. Compared with state-of-the-art methods, the proposed method achieved the best performance on two datasets, with F1 of 81.15% on the SYSU-CD dataset and 90.85% on the LEVIR-CD dataset.
论文介绍
为了解决“伪变化”的问题(即不同时间采集的遥感图像由于光照的不同,相同的物体或区域会呈现出不同的颜色或特征),文章结合卷积和Transformer提出了一种多尺度差分特征增强网络(Multi-scale difference feature enhancement network , MDFENet)。该网络中的差分增强模块包括多尺度差分增强编码器和Transformer解码器。它们应用于不同尺度的特征来建立双时图像特征的远程关系,增强语义变化的表示和不同尺度特征间的信息传递,减少“伪变化”。为缓解样本不平衡问题,引入多种损失的结合。与最先进的模型相比,它能获得更好的遥感图像变化检测效果。
Keywords
Change detection (CD), remote sensing, pseudo changes, feature enhancement, convolutional neural networks (CNNs), transformers, attention mechanism.
Facial expression recognition
方法框架
Illustration of the multi-scale difference feature enhancement network (MDFENet).
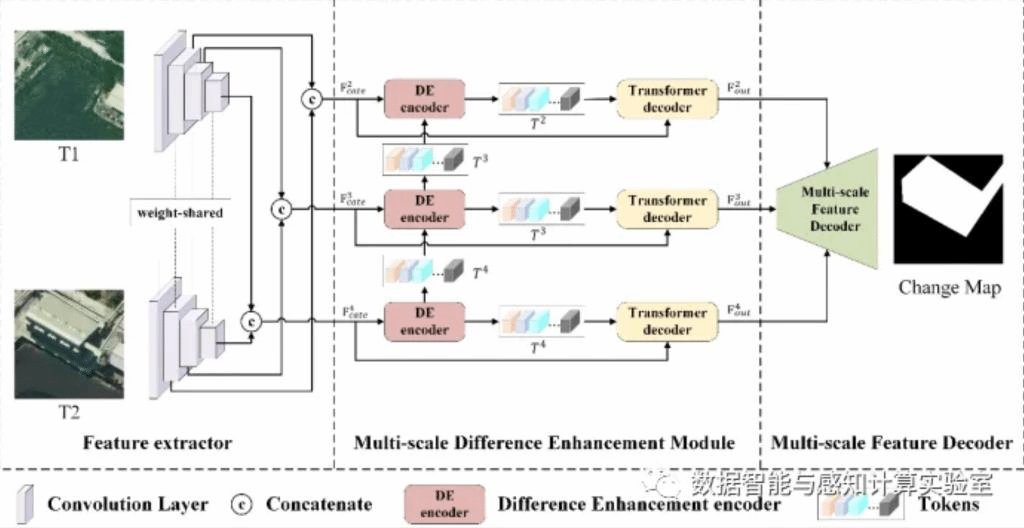
实验结果如下表:
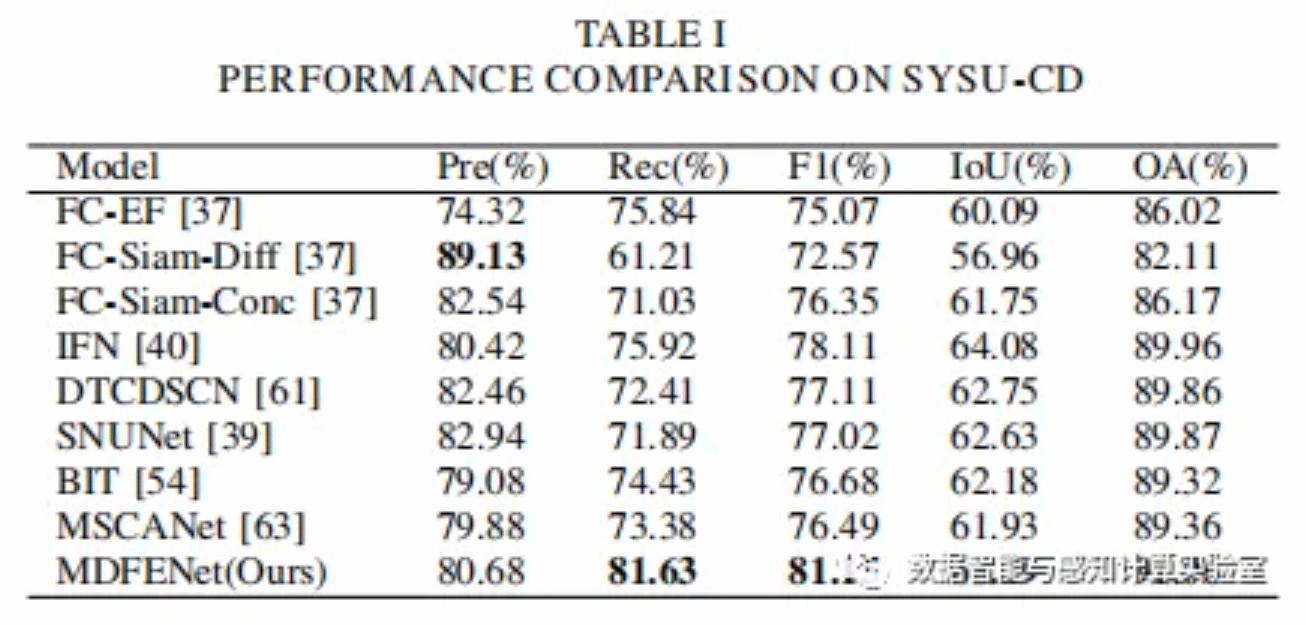
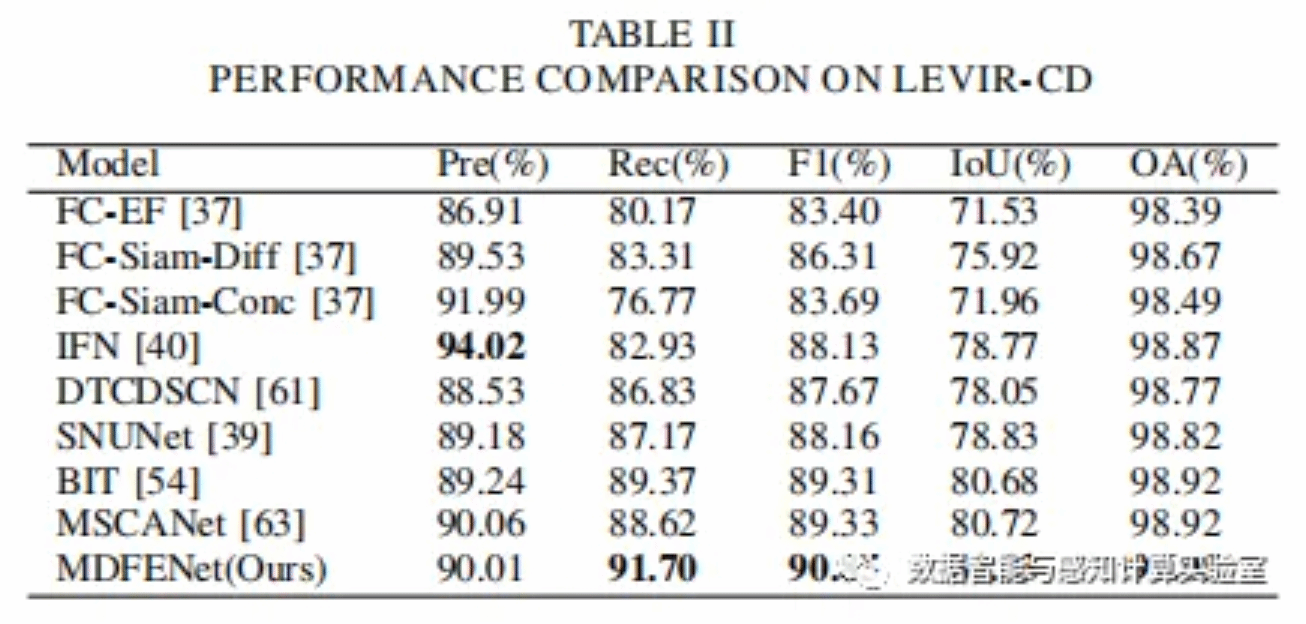
实验表明,本文构建的网络可以更好地从不同尺度进行特征增强,揭示了双时像图像的真实变化区域,减少了伪变化。