近日,我院教师研究论文被Remote sensing(IF:5.349,SCI二区top)期刊录用。论文的题目是H-RNet: Hybrid Relation Network for Few-Shot Learning-Based Hyperspectral Image Classification(http://doi.org/10.3390/rs15102497),以下是论文的简要分享:
Abstract
Deep network models rely on suffificient training samples to perform reasonably well, which has inevitably constrained their application in classifification of hyperspectral images (HSIs) due to the limited availability of labeled data. To tackle this particular challenge, we propose a hybrid relation network, H-RNet, by combining three-dimensional (3-D) convolution neural networks (CNN) and two-dimensional (2-D) CNN to extract the spectral–spatial features whilst reducing the complexity of the network. In an end-to-end relation learning module, the sample pairing approach can effectively alleviate the problem of few labeled samples and learn correlations between samples more accurately for more effective classifification. Experimental results on three publicly available datasets have fully demonstrated the superior performance of the proposed model in comparison to a few state-of-the-art methods.
论文介绍
近年来,深度学习已经在高光谱图像(HSI)分类中得到了广泛应用并取得了良好的效果。但是,深度学习模型通常需要大量标记样本才能实现最佳性能,而HSI由于人工标记难度大、耗时长,通常难以获得大量的标记样本,标记样本过少已成为深度学习算法应用于HSI分类问题中的一项重大挑战。为了缓解小样本所带来的挑战,本文提出了一个混合关系网络(H-RNet),通过结合三维(3-D)卷积神经网络(CNN)和二维(2-D)CNN来提取光谱空间特征,同时降低网络的复杂性。在一个端到端的关系学习模块中,样本配对方法可以有效地缓解标记样本少的问题,并更准确地学习样本之间的相关性,以实现更有效的分类。在三个公开的数据集上的实验结果充分证明了所提出的模型与一些最先进的方法相比的优越性能。
Keywords:
HSI classifification; few-shot learning; relation network; transfer learning
方法框架
General framework of H-RNet.
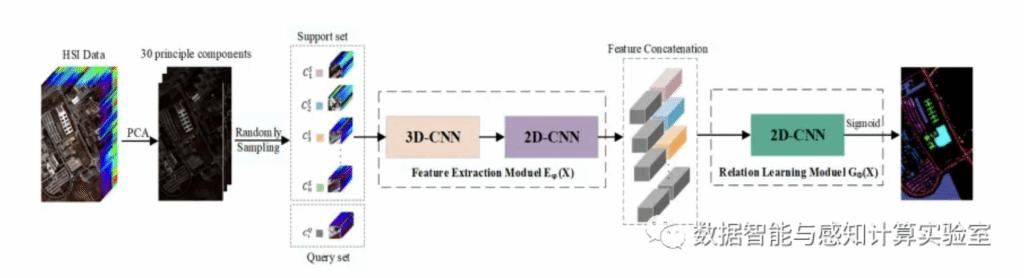
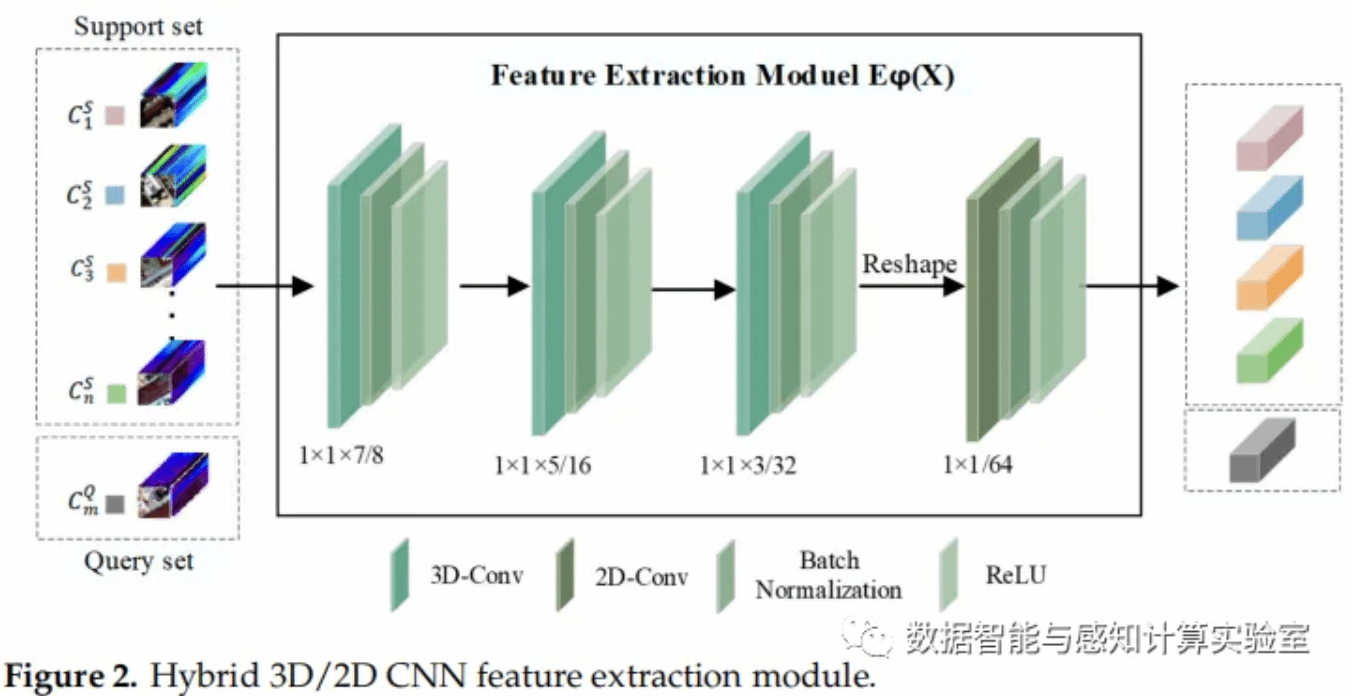
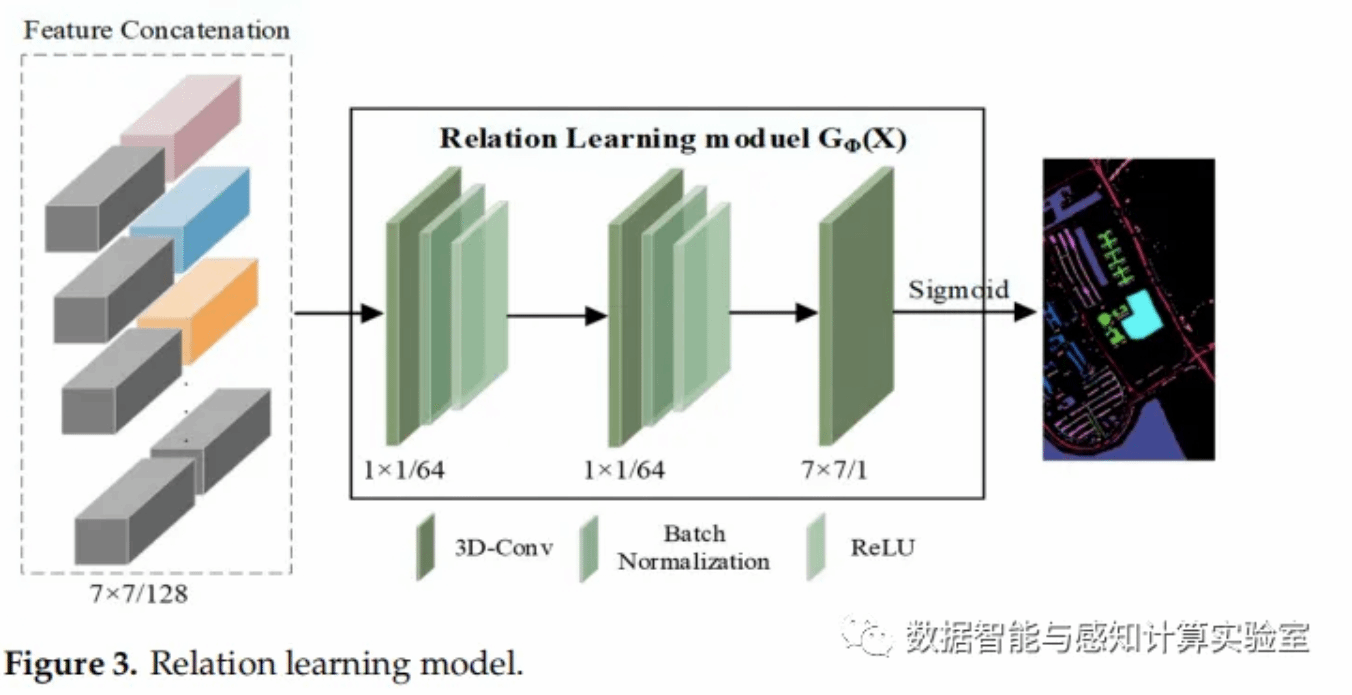
在三个数据集上的实验结果如下:
Pavia University (PU) dataset:
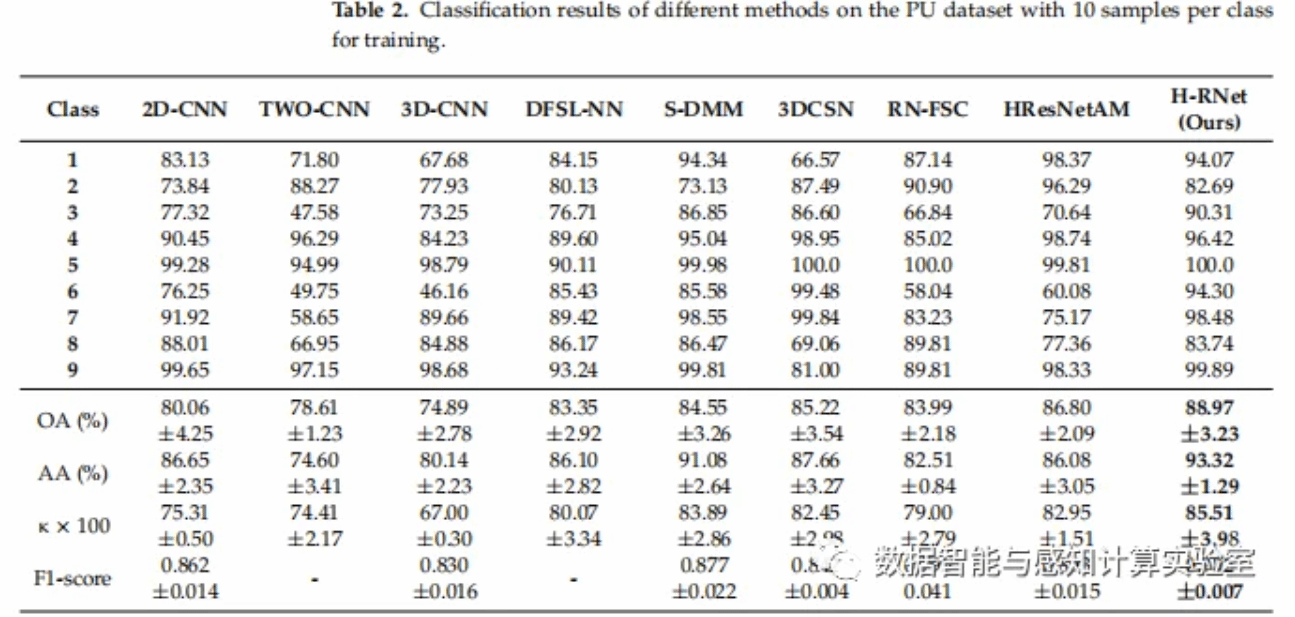
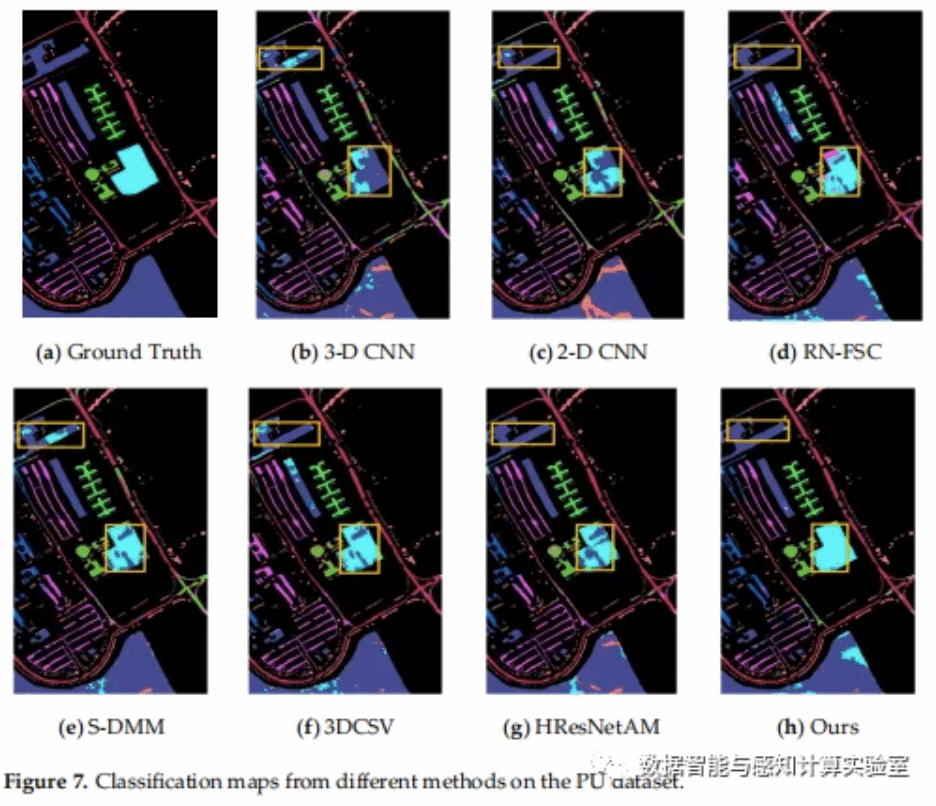
Salinas (SA) dataset:
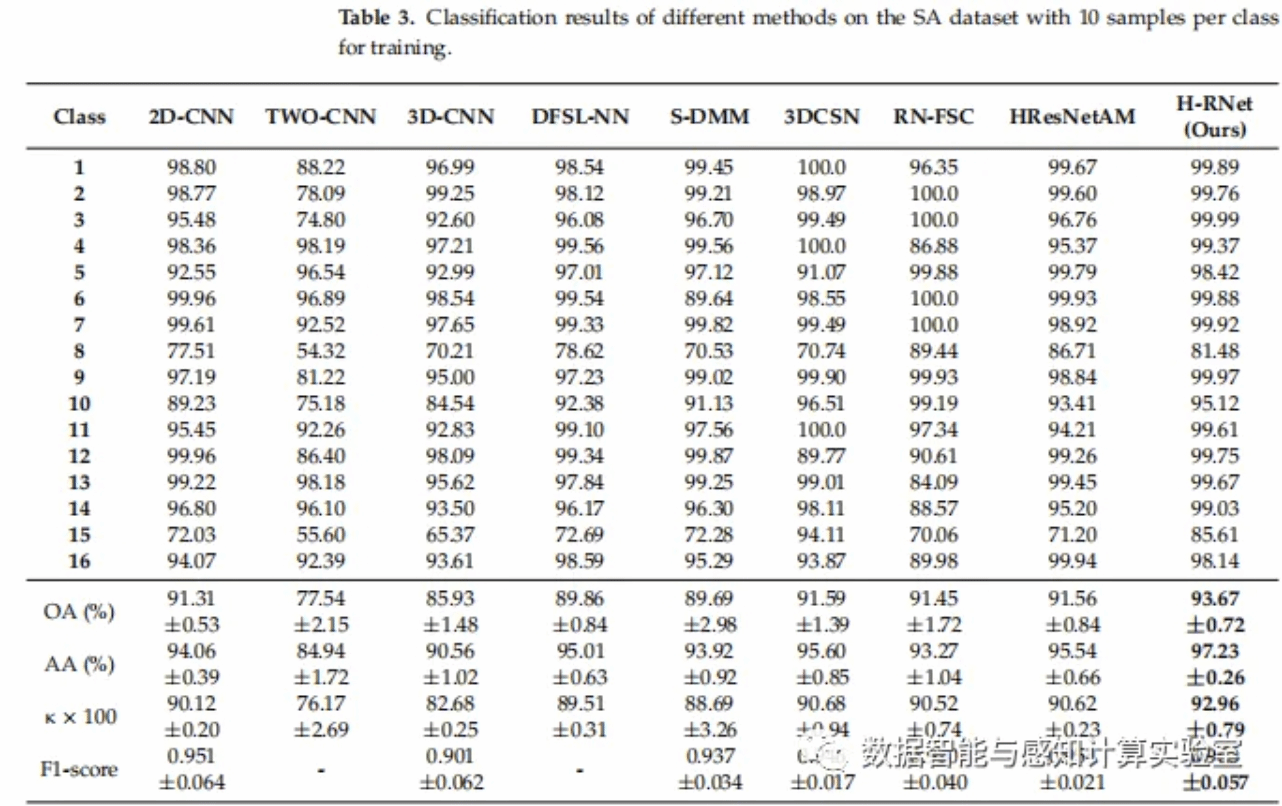
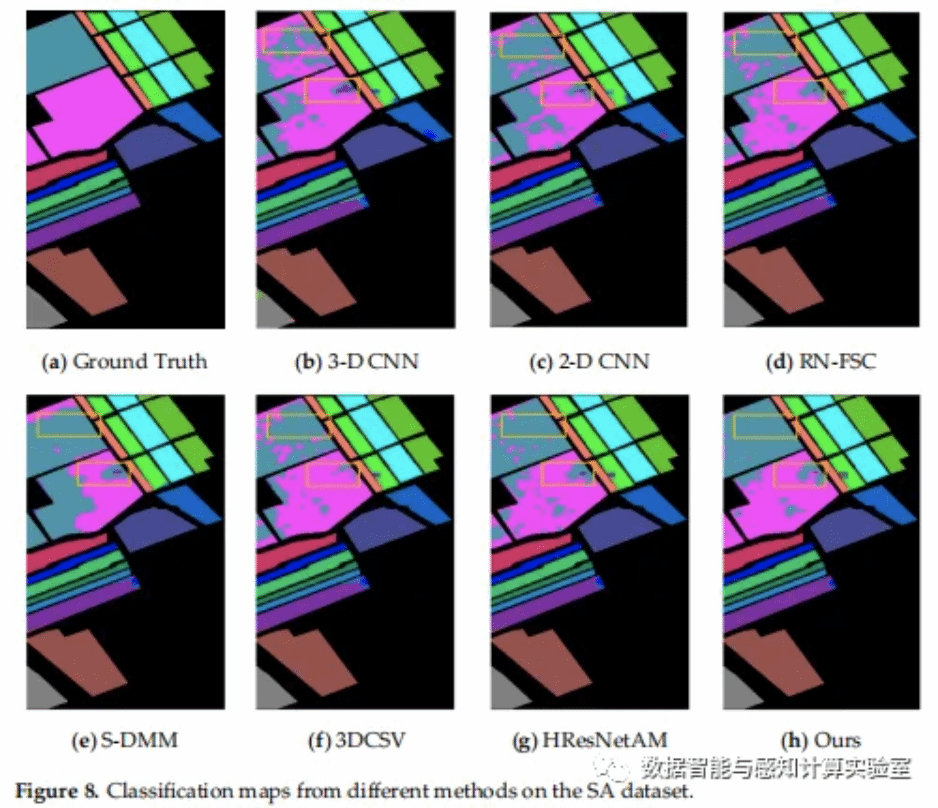
Pavia Centre (PA) dataset:
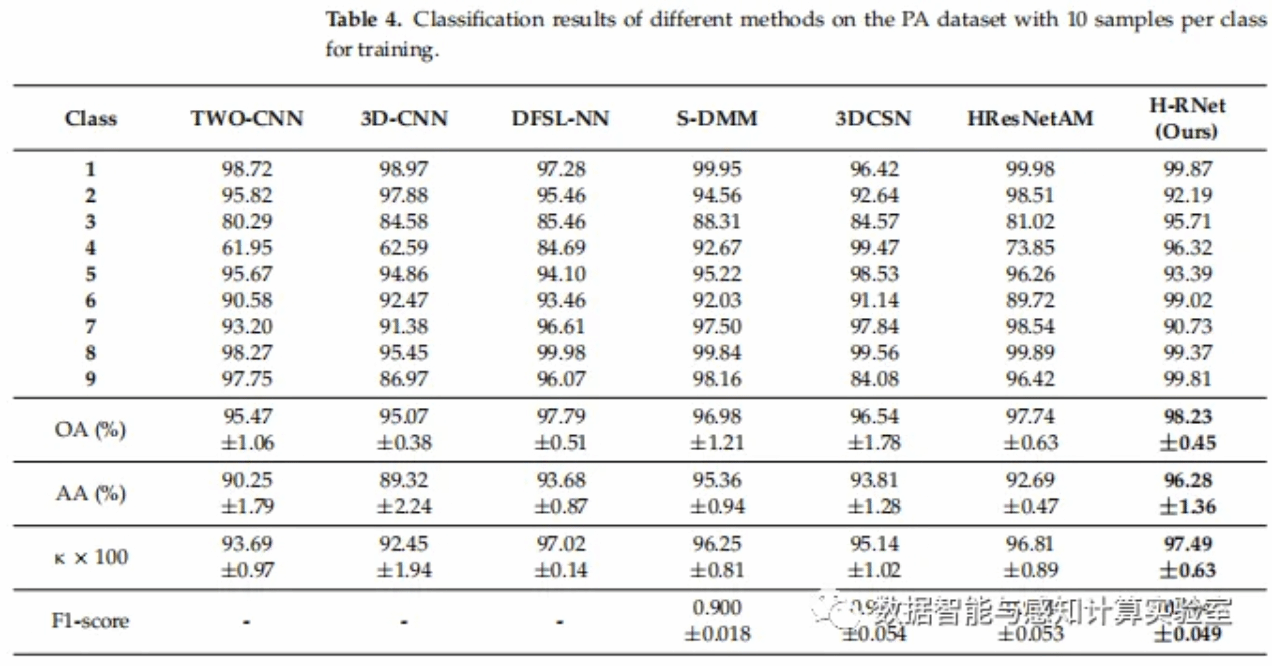
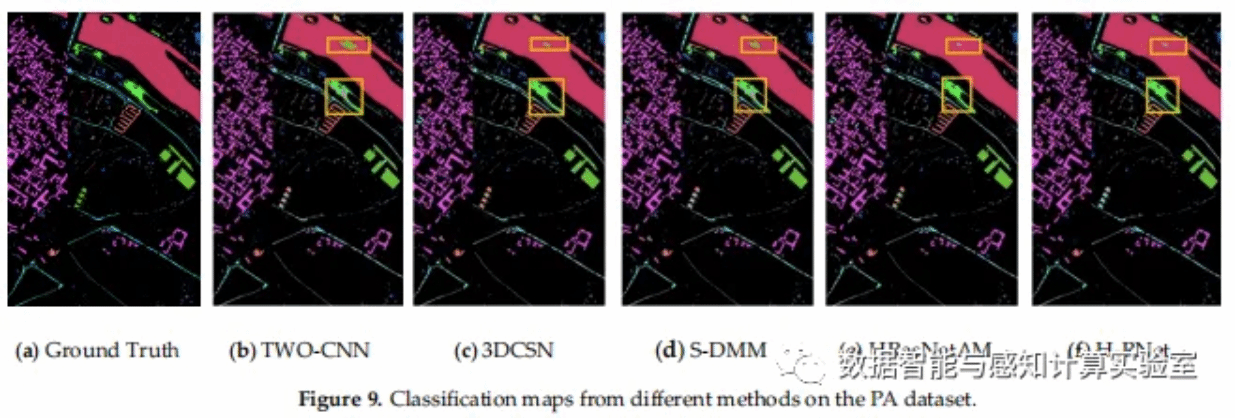
实验结果表明,本文的H-RNet方法可以更好地从高维数据中提取空间和光谱信息,在高维图像分类任务中具有更高的准确性和鲁棒性。